Course curriculum
The task of identifying and meeting the needs of any potential audience can be confounding, and data scientists and ML engineers are no exception. In order to reach wide success, any product that they use, be it an MLops platform or a small package, must do more than meet their needs. A product used by ML practitioners needs to also enable them to go even further in their efforts. With nearly 100 million hours of compute being provided to our users, we have been able to glean a number of lessons about the traits and patterns MLops platform users commonly hold. This talk is aimed to discuss and breakdown a subset of these behaviors, and through their analysis work towards identifying the cause, reasoning behind, and, in many cases, remedy for these typical behaviors and problems. Some of these patterns include: -Why it often makes sense to start in notebooks. -Why it’s so difficult to configure environments for ML. -Why reproducibility is so difficult to achieve. -Why it is often difficult to communicate findings in ML to non-practitioners and stakeholders. -Why it is so important to work with scalability in mind when working with ML. Using these subjects as our lens, we theorize on a unified design philosophy for MLops platforms meant to facilitate and help this same audience flourish. Attendees can expect to leave this talk with a renewed understanding of the challenges facing data scientists today, and how to apply these lessons to creating better products for them with these challenges in mind.
-
1
What We’ve Learned Pushing Nearly 100M Hours of GPU Compute
-
What We’ve Learned Pushing Nearly 100M Hours of GPU Compute
-
Instructor
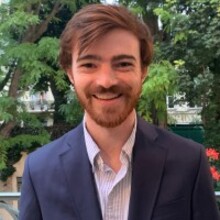
Technical Evangelist, Paper Space
James Skelton