Course curriculum
Machine learning (ML) systems are rapidly increasing in size, are acquiring new capabilities, and are increasingly deployed in high-stakes settings. As with other powerful technologies, safety for ML should be a leading research priority. In response to emerging safety challenges in ML, such as those introduced by recent large-scale models, I outline a roadmap for ML Safety and refine the technical problems that the field needs to address. I present three pillars of ML safety, namely withstanding hazards (Robustness), identifying hazards (Monitoring), and steering ML systems (Alignment).
-
1
Unsolved ML Safety Problems
-
Unsolved ML Safety Problems
-
Instructor
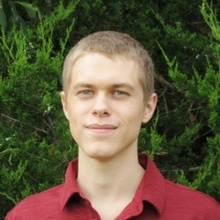
Research Intern,Deep Mind
Dan Hendrycks
Dan Hendrycks is a PhD candidate at UC Berkeley, advised by Jacob Steinhardt and Dawn Song. His research aims to disentangle and concretise the components necessary for safe AI. His research is supported by the NSF GRFP and the Open Philanthropy AI Fellowship. Dan has helped contribute the GELU activation function, the default activation in most Transformers including BERT, GPT, and Vision Transformers.