Course curriculum
Data Observability (DO) is an emerging category that proposes to help organizations identify, resolve, and prevent data quality issues by continuously monitoring the state of their data over time. This talk is a deep dive into DO, starting from its origins (why it matters), defining the scope and components of DO (what it is), and finally closing with actionable advice for putting observability into practice (how to do it). The first origin of DO is from software observability, a category that emerged in the 2010s to help companies gain visibility into software infrastructure by continuously collecting three pillars of information: metrics, traces, and logs. The second origin is from data quality, in which early pioneers like Richard Wang and Diane Strong laid the foundations for describing for describing the different aspects of data that should be monitored in order to ensure its quality. With these origins in place, we’ll then rigorously define data observability to understand why it is different from software observability and existing data quality monitoring. We will derive the four pillars of DO (metrics, metadata, lineage, and logs) then describe how these pillars can tied to common use cases encountered by teams using popular data architectures, especially on cloud data stacks. Finally, we’ll close with pointers for how to put observability into practice, drawing from our experience helping teams across sizes, from fast-growing startups to large enterprises, successfully implement DO. Successfully implementing observability throughout an organization involves not only using the right technology, whether that be a commercial solution, an in-house initiative, or an open source project, but implementing the correct processes with the right people responsible for specific jobs.
-
1
The Origins, Purpose, and Practice of Data Observability
-
The Origins, Purpose, and Practice of Data Observability
-
Instructor
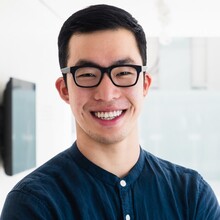
Co-founder and CEO Metaplane
Kevin Hu