COURSE ABSTRACT
Artificial intelligence is making its way into budgets at enterprises and startups alike. Companies are ramping up on investments in AI and machine learning in the hopes of transforming their business with automated insights based on algorithms. But are they prepared to implement AI the right way? As AI implementations reach a broader set of companies, there are important lessons to be learned on how to avoid algorithms that are inherently biased, or that will make unethical or immoral conclusions based on skewed or misleading data.
Though science is known as the objective truth, the people who code algorithms, and the data sources they choose to build them from, should not be held in the same regard. Coders may have implicit or explicit biases that show up in their work, creating data that is in effect biased and could breed immoral outcomes in the rapidly approaching expansion of AI. Because of this, AI/ML professionals, data scientists, and business leaders must ensure the algorithms they are creating are handled cautiously and thoughtfully, with consideration of the biases they may condone. Indeed, historically biased data can produce automation of that data that can create issues of racism, sexism, or many other combinations of group favoritism.
In this session, Harry Glaser, President, Data Business & GM, San Francisco at Sisense, will draw on experiences working with 1,000+ data teams to dissect how data scientists and AI/ML professionals can make sure they implement AI the right way—rid of potential sources of bias. He will discuss common sources of bias, how to ensure readiness early on with best practices for generating data, and the value companies can see as a result.
-
1
Sources of Bias: Strategies for Tackling Inherent Bias in Ai - Harry Glaser
-
On-Demand Recording
-
INSTRUCTOR
Instructor Bio
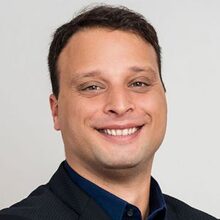
President, Data Business & GM | Sisense
Harry Glaser