Course Curriculam
Machine learning has been hastily operationalised, often with little regard for its wider societal impact. At the same time, there's been a lack of clear, concrete guidelines on how to reduce the risks stemming from AI. With that in mind, we have started a Non-profit organization, the Foundation for Best Practices in Machine Learning. Our goal is to help data scientists, governance experts, managers, and other machine learning professionals implement ethical and responsible machine learning. We do that via our free, open-source technical and organisational Best Practices for Responsible AI.
These guidelines have been developed principally by senior ML engineers, data scientists, data science managers, and legal professionals. The Foundation’s philosophy is that (a) context is key, and (b) responsible ML starts with prudent MLOps and product management.
The technical and organisational best practices look at both the technical and institutional requirements needed to promote responsible ML. Both blueprints touch on subjects such as “Fairness & Non-Discrimination”, “Representativeness & Specification”, “Product Traceability”, “Explainability” amongst other topics. Where the organisational guide relates to organisation-wide process and responsibilities (f.e. the necessity of setting proper product definitions and risk portfolios); the model guide details issues ranging from cost function specification & optimisation to selection function characterisation, from disparate impact metrics to local explanations and counterfactuals. It also addresses issues concerning thorough product management.
Instructors

Data Scientist ABN Amro Bank
Violeta Misheva
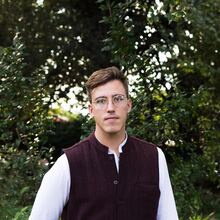
Legal Counsel for AI & Data Science
Daniel Vale