Live training with Aric LaBarr starts on April 20th at 1 PM (ET)
Training duration: 4 hours (Hands-on)
Instructor Bio:
Aric LaBarr, PhD
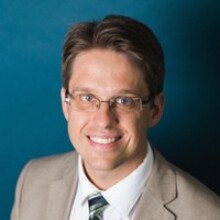
Associate Professor of Analytics | Institute for Advanced Analytics at NC State University
Aric LaBarr, PhD
30% discount ends in:
-
00 Days
-
00 Hours
-
00 Minutes
-
00 Seconds
By the end of the course, participants will be able to:
-
Use network analysis to create good features for fraud models like centrality and connectivity
-
Properly oversample or undersample a rare event data set as well as use synthetic sampling techniques like SMOTE
-
Build a supervised fraud classification model using one of the following: logistic regression, tree based algorithms, and naive Bayes models
-
Build a supervised NOT-fraud classification model using one of the above techniques
-
Interpret a complicated model using LIME
Course Abstract
Course Outline
1. Review of Fraud
- The Problem of Fraud - How can we analytically define fraud? There are important characteristics of fraud that puts a better perspective on the modeling and identification of fraud.
- Detection and Prevention - The two biggest pieces that any holistic fraud solution should have are detection of previous instances of fraud and prevention of new instances. This section also defines the typical fraud identification process in organizations.
- Analytical Solution - Now that we now what fraud is as well as the organizational structure of how to deal with fraud, we need to introduce the analytical approaches to becoming a mature organization on detecting and preventing fraud.
2. Data Preparation
- Review of Feature Engineering - The best way to glean information from data is to develop good features to help detect and identify fraud. We talk about and develop strategies for developing good features for anomaly detection. Briefly review RFM Features and categorical feature creation as well.
- Introduction of Network Approaches - When generating features, we can also incorporate the ideas of network analysis to our modeling framework. Who people are connected to could play a major role in detecting instances of fraud as well as complex fraud rings.
- Obtaining Labeled Data - The hardest part about modeling fraud is obtaining labeled cases of fraud. In this section we will talk about using anomaly models, subject matter experts, and/or unsupervised techniques to obtain labels for suspected fraud.
- Sampling Concerns - Fraud is typically and hopefully a rare event at a company. However, this poses problems for modeling. In this section we cover the process of oversampling and undersampling to account for rare event modeling problem. We also introduce the Synthetic Minority Oversampling TEchnique (SMOTE).
3. Supervised Fraud Models
- Classification Scoring - This section reviews the concepts of classification models and how they are used to rank and score observations for fraud.
- Logistic Regression - This section reviews the concept of logistic regression which is a more statistical based and interpretable model for fraud detection.
- Tree-Based Algorithms - This section covers the concepts of tree based models. It starts with focusing on decision trees and their generalization to random forests. We then introduce the concepts of gradient boosting approaches without going into too much mathematical detail.
- Naive Bayes Model - The naive Bayes model is a great model to use for fraud detection. This section introduces the main ideas and uses for the naive Bayes model.
- Supervised NOT-Fraud Model and Model Evaluation - The previous techniques all focus on previous instances of fraud and detecting those again. Here we talk about the important process of identifying new instances of fraud we haven't seen before using the NOT-Fraud model and combining it with the fraud model. We also discuss how to properly evaluate your fraud models.
4. Clustering and Implementation
- Clustering of Scored Observations - Once you have an idea about which observations don't look like either previous instances of fraud or not fraud, how do you isolate and investigate these? We need to use clustering to help isolate groups of observations that might identify new types of fraud.
- Interpretability - The people who are typically investigating cases of fraud are not the data scientists who build the models. With this being the case, we need to make sure our models are interpretable and implementable for easy use by investigators by using scorecards or Local Interpretable Model Explanations (LIME).
- Long-term Fraud Strategy - To wrap-up our discussion on fraud, we talk about how to continue to implement these pieces into the grander fraud framework at a company. We also talk about how to evaluate an entire fraud system, not just the models themselves, after the models have been introduced.
Which knowledge and skills you should have?
-
Introductory R/Python
-
Basic introduction to supervised modeling
-
Basic introduction to classification models like logistic regression, decision trees, etc. (this isn't required, but helpful for understanding)
What is included in your ticket?
-
Access to live training and QA session with the Instructor
-
Access to the on-demand recording
-
Certificate of completion