Course curriculum
There is a pressing need for tools and workflows that meet data scientists where they are. This is also a serious business need: How to enable an organisation of data scientists, who are not software engineers by training, to build and deploy end-to-end machine learning workflows and applications independently. In this talk, we discuss the problem space and the approach we took to solving it with Metaflow, the open-source framework we developed at Netflix, which now powers hundreds of business-critical ML projects at Netflix and other companies from bioinformatics and drones to real estate. We wanted to provide the best possible user experience for data scientists, allowing them to focus on parts they like (modeling using their favorite off-the-shelf libraries) while providing robust built-in solutions for the foundational infrastructure: data, compute, orchestration, and versioning. In this talk, you will learn about: 1. What to expect from a modern ML infrastructure stack. 2. Using tools such as Metaflow to boost the productivity of your data science organisation, based on lessons learned from Netflix and many other companies. 3. Deployment strategies for a full stack of ML infrastructure that plays nicely with your existing systems and policies.
-
1
Human-Friendly, Production-Ready Data Science with Meta flow
-
Human-Friendly, Production-Ready Data Science with Metaflow
-
Instructor
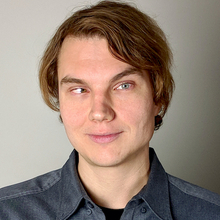
Co-Founder Outerbounds
Ville Tuulos